RAG: Benifits of RAG
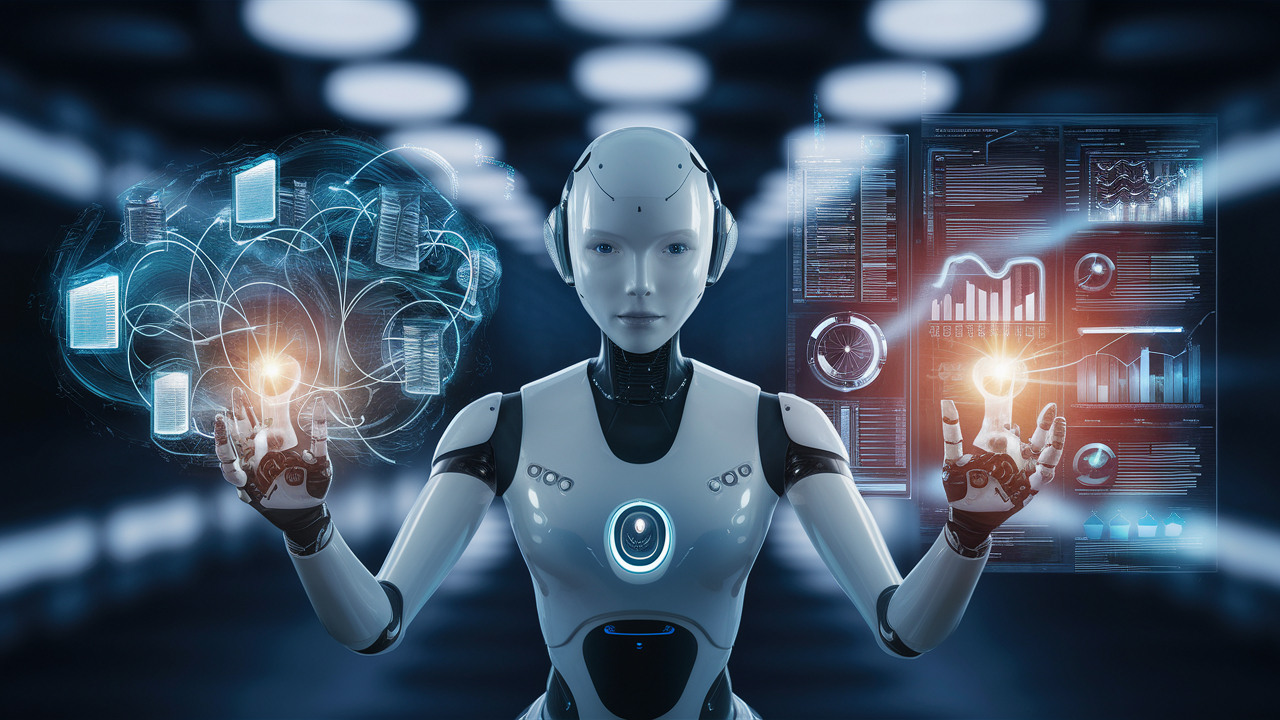
Unlocking the Power of RAG in Enterprise AI
As technology is developing rapidly companies always look for new ways to use AI tech to make better choices and work more. One new technology that's grabbing attention in the tech world is Retrieval Augmented Generation (RAG). In this post, we'll look at what RAG is, how people use it, and why it's providing a new aspect for companies in many fields.
What is Retrieval Augmented Generation (RAG)?
RAG is an AI method that combines the strength of large language models (LLMs) with the ability to find and add useful information from outside data sources. You can think of it as a smart AI system that can tap into a huge knowledge database.
The Technological Problems RAG Solves:
Retrieval Augmented Generation (RAG) addresses several critical challenges in AI and natural language processing:
-
Hallucination and Accuracy: Traditional large language models can sometimes generate reasonable sounding but incorrect information, a term known as "hallucination." RAG mitigates this by grounding responses in verified, external data sources, significantly improving accuracy.
-
Knowledge Cutoff: Large language models are trained on data up to a certain date, after which they can't access new information which limits their functionality. RAG solves this by allowing real-time access to up-to-date information, keeping responses current and relevant.
-
Domain-Specific Knowledge: While general-purpose language models have broad knowledge, they may lack depth in specialized fields. RAG allows the integration of domain-specific knowledge bases, enabling more precise and relevant responses in specialized contexts.
-
Data Privacy and Control: Organizations often need to use proprietary or sensitive information that can't be included in public AI models. RAG enables the use of private, controlled data sources, allowing businesses to leverage their own information securely. Thus, providing a layer of security over the organization's data.
-
Scalability of Knowledge: Continuously retraining large language models to include new information is resource-consuming and impractical. RAG provides a more efficient way to expand an AI system's knowledge by simply updating the external knowledge base.
-
Explainability and Transparency: In many industries, it's crucial to understand how AI systems arrive at their conclusions. RAG improves explainability by providing clear sources for the information used in generating responses.
-
Contextual Understanding: RAG enhances an AI system's ability to understand and respond to queries in the correct context by providing relevant background information from the knowledge base.
-
Multilingual and Cross-Domain Applications: By leveraging external knowledge sources, RAG can more effectively handle queries across different languages and domains, even if the base language model wasn't extensively trained in these areas.
-
Reducing Bias: While not eliminating bias entirely, RAG can help reduce it by incorporating diverse, verified and authenticated information sources, rather than relying solely on the training data of the language model.
-
Cost-Effective Customization: Instead of fine-tuning entire language models (which can be extremely costly), RAG allows for cost-effective customization of AI capabilities by simply updating or changing the external knowledge base. Thus, making it pocket friendly.
By addressing these technological challenges, RAG opens new possibilities for AI applications in enterprise settings, enabling more accurate, current, and trustworthy AI-driven solutions.
The Benefits of RAG for Companies
-
Correctness: RAG has a big impact on cutting down AI mistakes or old information by basing answers on checked facts.
-
Personalization: Companies can use their own private data sources making sure the AI's knowledge fits their exact needs and field. Thus, catering to the company's requirements and needs.
-
Growth: RAG systems can handle lots of different questions across many areas, making them useful tools for various teams in a company.
-
Clarity: Unlike old "black box" AI models, RAG shows where information comes from boosting trust and making things easier to understand.
- Real-time Updates: When the knowledge base gets new information, the RAG system's answers change right away. This happens without having to retrain the whole model.
How Does RAG Work?

-
Query Processing: The system looks at what the user asks to figure out what information it needs to find.
-
Information Retrieval: Then, it searches its knowledge base (often kept in a vector database) to find relatable information.
-
Context Augmentation: It mixes the information found with the original question to make a detailed prompt.
-
Generation: This augmented prompt is fed into the large language model, which generates a response based on both its training and the retrieved information.
Output: The system provides an answer that's not only logical but also well balanced in up-to-date, relevant data.
Conclusion
Retrieval Augmented Generation represents a significant leap forward in Artificial intelligence capabilities. By combining the creative power of large language models with the accuracy of curated knowledge databases, RAG offers businesses a powerful tool for enhancing decision-making, improving customer experiences, and driving innovation.
For businesses looking to stay ahead in the race of artificial intelligence and those who want to take a leap in the advancement of technology, implementing RAG could be the key to unlocking new levels of productivity and innovation.
Ready to explore how RAG can transform your business? Contact our team of AI experts today to learn more about implementing this cutting-edge technology in your organization.